Integrating molecular and epidemiological data to forecast high-risk viral variants and guide pandemic response strategies
The rapid evolution of SARS-CoV-2 variants during the COVID-19 pandemic emphasized the need for advanced tools to predict future outbreaks. The study introduces CoVFit, an AI-driven model that analyzes spike protein mutations to assess the fitness of SARS-CoV-2 variants. By combining molecular and epidemiological data, CoVFit provides early insights into the transmissibility and immunity evasion of emerging variants, offering a powerful tool for pandemic preparedness, vaccine design, and global risk assessment.
Viral infectious diseases pose significant challenges due to the rapid evolution of viruses through mutations. This was particularly evident during the COVID-19 pandemic, when emerging variants of SARS-CoV-2 sparked new waves of infection. These variants often carry mutations that made them more transmissible, allowing them to spread rapidly across populations. Understanding a virus’s "fitness"—its ability to spread in a host population—has become essential for managing and anticipating viral threats. Though there are methods to assess the fitness of variants based on mutation patterns, statistical models that consider interactions between mutations are lacking.
To address this challenge, a team of researchers led by Associate Professor Jumpei Ito, including Dr. Adam Strange and Professor Kei Sato, from The Institute of Medical Science at The University of Tokyo, Japan, introduced CoVFit, a novel framework designed to predict the fitness of SARS-CoV-2 variants. Their findings were published online in Nature Communications on May 13, 2025. CoVFit integrates molecular data with large-scale epidemiological data to provide a predictive model that helps us understand why some variants succeed while others do not. This framework offers more than just tracking the spread of the virus; it reveals the underlying reasons for its success, making it a powerful tool for real-time surveillance and response in the face of ongoing and future viral outbreaks.
The CoVFit model was developed through an innovative approach that combined molecular and epidemiological data. The team focused on mutations in the spike (S) protein, which affect the virus’s ability to escape immune protection from past infections or vaccinations, alongside population-level trends like variant prevalence over time and in different regions. By combining this information, CoVFit was trained and tested to predict a variant’s fitness score.
Dr. Ito explains, “We developed an artificial intelligence (AI) model, CoVFit, that predicts the fitness of SARS-CoV-2 variants based on the S protein sequence. Using CoVFit, we elucidated which mutations SARS-CoV-2 has acquired to enhance its fitness and repeatedly expand its spread.” The model demonstrated an impressive ability to predict the evolutionary impact of single amino acid substitutions in the virus with high accuracy, offering insights into how the virus evolves and spreads. Dr. Ito also notes, “CoVFit is expected to enable the early detection of high-risk variants with a high potential for widespread transmission, ideally at the point when just a single sequence of the variant is registered in a database.”
The team further developed a prospective approach to forecast viral evolution using CoVFit. They systematically generated in silico mutant variants by introducing all possible single amino acid substitutions into a reference strain and predicted the fitness of each. This enabled the identification of mutations with a high likelihood of emerging in future variants. When applied to the Omicron BA.2.86 lineage, CoVFit predicted that substitutions at S protein positions 346, 455, and 456 would enhance viral fitness. Remarkably, these exact mutations were later observed in BA.2.86 descendant lineages—JN.1, KP.2, and KP.3—that subsequently spread globally. Dr. Ito concludes, “These findings underscore CoVFit’s ability to anticipate evolutionary changes driven by single amino acid substitutions.”
In conclusion, CoVFit represents a major breakthrough in our ability to predict, interpret, and respond to viral evolution. By integrating molecular biology with population-level data through AI, it provides a flexible, transparent, and timely approach to pandemic preparedness. As viruses continue to evolve, tools like CoVFit will play a critical role in guiding proactive and informed public health responses worldwide.
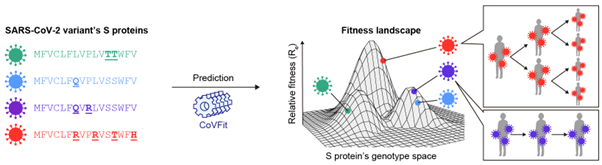
CoVFit can predict the epidemic potential—i.e., the fitness—of SARS-CoV-2 variants based solely on their spike protein sequences.
Reference
Journal:Nature Communications
Title of original paper:
A protein language model for exploring viral fitness landscapes
DOI:
10.1038/s41467-025-59422-w
Authors:
Jumpei Ito1,2, Adam Strange1, Wei Liu1,3,4, Gustav Joas1,5,
Spyros Lytras1,6, The Genotype to Phenotype Japan (G2P-Japan) Consortium, and Kei Sato1,2,6,7
Affiliations:
1Department of Microbiology and Immunology, The University of Tokyo, Tokyo, Japan
2International Research Center for Infectious Diseases, The University of Tokyo, Tokyo, Japan
3Department of Genetic Medicine and Development, University of Geneva, Geneva, Switzerland
4Swiss Institute of Bioinformatics, Geneva, Switzerland
5Department of Medicine, Karolinska Institutet, Stockholm, Sweden
6Centre for Virus Research, University of Glasgow, Glasgow, UK
7International Vaccine Design Center, The University of Tokyo, Tokyo, Japan
About The Institute of Medical Science, The University of Tokyo
The Institute of Medical Science, The University of Tokyo (IMSUT), established in 1892 as the Institute of Infectious Diseases and renamed IMSUT in 1967, is a leading research institution with a rich history spanning over 127 years. It focuses on exploring biological phenomena and disease principles to develop innovative strategies for disease prevention and treatment. IMSUT fosters a collaborative, interdisciplinary research environment and is known for its work in genomic medicine, regenerative medicine, and advanced medical approaches like gene therapy and AI in healthcare. It operates core research departments and numerous specialized centers, including the Human Genome Center and the Advanced Clinical Research Center, and is recognized as Japan’s only International Joint Usage/Research Center in life sciences.About Jumpei Ito from the Institute of Medical Science, The University of Tokyo
Dr. Jumpei Ito is an Associate Professor in the Department of Systems Virology, Institute of Medical Science, The University of Tokyo. He holds a Ph.D. in Science from the Graduate University for Advanced Studies. His research focuses on viral evolution, epidemic prediction, and AI-driven infectious disease control. His expertise spans virology, bioinformatics, machine learning, and veterinary science. He has authored numerous publications and received several honors, including the MEXT Young Scientists' Prize, and actively mentors young researchers in the field of virus informatics.
Funding information
This study was supported in part by JST PRESTO (JPMJPR22R1, to J.I.); JSPS KAKENHI Grant-in-Aid for Scientific Research B (25K00116, to J.I.); JSPS KAKENHI Grant-in-Aid for Early-Career Scientists (23K14526, to J.I.); AMED SCARDA Japan Initiative for World-leading Vaccine Research and Development Center “UTOPIA” (JP223fa627001 and JP243fa627001h0003, to J.I.; JP223fa627001 and JP243fa627001h0003, to K.S.); SHIONOGI Infectious Disease Research Promotion Foundation (to J.I.); AMED ASPIRE Program (JP24jf0126002, to G2P-Japan Consortium and K.S.); AMED Research Program on Emerging and Re-emerging Infectious Diseases (23fk0108583, 24fk0108690,24wm0225041, to K.S.); JSPS KAKENHI Fund for the Promotion of Joint International Research (International Leading Research) (JP23K20041, to G2P-Japan Consortium and K.S.); JSPS KAKENHI Grant-in-Aid for Scientific Research A (JP24H00607, to K.S.); Mitsubishi UFJ Financial Group, Inc. Vaccine Development Grant (to Jumpei Ito to K.S.); and The Cooperative Research Program (Joint Usage/Research Center program) of Institute for Life and Medical Sciences, Kyoto University (to K.S.); JSPS International Research Fellow (to G.J.).Media contact
Affiliation: Project Coordination Office, The Institute of Medical Science, The University of Tokyohttps://www.ims.u-tokyo.ac.jp/